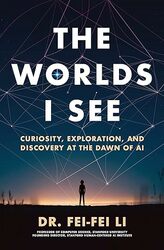
Rating: 7.6/10.
The Worlds I See: Curiosity, Exploration, and Discovery at the Dawn of AI by Fei-Fei Li
A fairly easy-to-read memoir by Fei-Fei Li, a computer vision researcher most well-known for her work on ImageNet. The first half of the book discusses her childhood, focusing on her experiences as an immigrant to America, while the second half of the book focuses more on her scientific achievements and her role in the early years of the deep learning revolution.
Fei-Fei was raised in Chengdu, China, in a middle-class family with intellectual parents. Her father immigrated to the US in 1989, and she and her mother came to the US three years later when she was 15 years old, settling in New Jersey. In her early years at high school in New Jersey, she excelled in math but struggled with English. She worked at a restaurant to help the family financially and made friends with her math teacher, Mr. Sabella, who turned out to be a lifelong friend. She was accepted to Princeton, where she studied physics and gained her first research experience in computational neuroscience, enjoying the process so much that she turned down a more lucrative job in finance to pursue what she loved most: research. She then went to grad school at Caltech, where she studied computer vision.
Fei-Fei’s earliest research at Caltech focused on human visual information processing, such as decomposing the steps and measuring how long it takes for humans to recognize objects without paying conscious attention. She also conducted computational work on computer vision methods to recognize categories with just one example. During that time, there was a lot of focus on algorithmic methods, but she realized that datasets were more important than algorithms. She understood that more could be gained by collecting more data and more categories of images, as prior work only had a small number of categories. This realization led her to start collecting ImageNet, which has thousands of categories and 1,000 examples per category. This was a huge effort involving lots of scraping and crowdsourced labels from Mechanical Turk. Although it was accepted at CVPR 2009, it was relatively ignored. This changed when they organized it into a competition to see who could create the best models for image recognition on the ImageNet dataset. The first two years were relatively disappointing as the winners used SVMs, which were conventional techniques that had been around for a long time. In 2012, AlexNet won by a wide margin using neural networks, something that had been considered dead for decades.
After the success of neural networks in image recognition, the field of deep learning quickly exploded as researchers extended the technique to numerous applications, such as fine-grained image classification to identify car models and image captioning by combining vision and language. The work progressed rapidly, with the same experiments often being conducted by two teams in parallel as the field grew so quickly. The author also began some projects to detect hand washing in hospitals, which led to ethical questions about the automated monitoring of healthcare staff. Some nurses pushed back against the technology, and she realized the human aspect of technology as she was simultaneously a computer vision AI for healthcare researcher while her mother was receiving treatment for a terminal illness in a hospital. The field advanced quickly to where we are now, with lots of new advances every year in self-driving cars and game-playing AI like AlphaGo. The author eventually joined Google to lead a research team there.