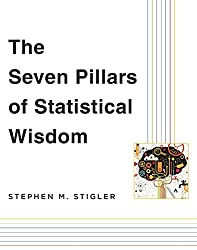
Rating: 7.7/10.
Book about the history of statistics, grouped into seven “pillars” – key ideas that unify modern statistics as a discipline. Each of the seven chapters has catchy titles: Aggregation, Information, Likelihood, Intercomparison, Regression, Design, and Residual. Many key ideas seems obvious in retrospect, but it took a surprisingly long time before anyone thought to try them. The aggregation chapter is about combining multiple data points into a single value, such as taking the average, with the point being that the aggregated value is more accurate than any individual observation. Although ubiquitous today, this operation was historically uncommon; the common practice was to choose one observation as the best one. The book gives some examples of aggregating information to determine the position of magnetic north, and the size of the earth.
The information chapter is about quantifying how much more information we gain about a variable by collecting more data; it turns out that the accuracy of the measured quantity increases proportionally to the square root of the sample size, therefore, after some data has been collected, further data is of relatively less value. A historical scheme to detect coin fraud by weighing turned out to be too generous to the fraudsters because they did not understand this law. The likelihood chapter explores the development of statistical testing and p-values: how unlikely is the random observed pattern if it was purely due to random chance? A simple example was in examining the ratio of male to female births: a city reported for 84 consecutive years that there were more male than female births, so the likelihood of this happening randomly is one in 2^82. This was this idea was then expanded into trickier cases where it is less clear what is the criterion for event being unlikely.
The next few chapters explores more key developments in statistical history, although there’s less clear of a connection between the topic and chapter title. Pillar 4 discusses the Student t-test, where the population variance is unknown and must be influenced from the data while comparing two samples. Pillar 5 discusses Galton’s experiments on the physical variation between successive members of a family, and the apparent paradox when you try to explain why the variation doesn’t increase generation after generation. The key to this puzzle is realizing that the physical characteristic is the additive combination of a genetic component and a random component, where only the genetic component is passed down to the next generation, Thus creating a “regression to the mean” effect.
Pillar 6 discusses randomization of experiments and how when randomization is added to the beginning of experiments, it makes things easier to work with later. Finally, the 7th pillar discusses residuals, which are useful for comparing how well do model assumptions fit the data, and comparing two (often nested) models.
Overall, this is a good exploration of how key ideas in modern statistics were first discovered and the historical contexts where they were applied. There are many diagrams and excerpts from historical papers, which was pretty cool. A similar book about the history of statistics is “The Lady Tasting Tea”, this book is more focused on technical details of experiments, while the other book has more focus on the lives and personalities of important scientists. However, the author presented too many loosely-connected experiments with not enough details to fully appreciate them, especially in the later chapters. I could usually follow the gist of the experiment and how it’s connected to the modern statistics key idea, but not the technical details of the experiment, especially since they used different terminology and notation back then.