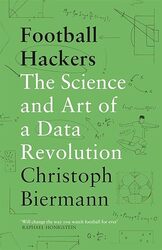
Rating: 7.7/10.
Book exploring the efforts of data scientists to analyze top-level football, primarily focusing on European teams, and how they use data and statistics to gain small advantages for their teams. One of the difficulties in analyzing football with data is that many ratings are subjective, determined by a sport commentator’s or journalist’s opinion rather than any objective measure. Additionally, the fact that football is a low-scoring game means that the issues of small sample size and luck play a significant role, leading people to rely on narratives and fall prey to confirmation bias rather than carefully analyzing data.
The expected goals (XG) metric represents the expected number of goals based on the positions of shots taken. It was one of the earliest metrics to be widely used after it was discovered that the probability of a goal did not vary much by the player. Instead, top players do not score more often from the same positions but rather create more goal-scoring chances, which somewhat mitigates the problems of small sample size since football is such a low-scoring game. However, even though there are many more attempts at shots than goals, the role of luck remains significant, and a team with a high XG may still lose. Coaches are often blamed when they have bad luck. Some early adoptors of data science include Benham and Anderson who started with football betting, and around 2014, they became coaches for a Danish football team, though they were outsiders, they applied mathematical models to make decisions on the field.
Conventional wisdom suggests that the best team simply spends more to acquire the best players. However, some coaches attempt to break norms and perform better with less skilled players. Some examples include Tuchel’s strategy of converging on the middle and avoiding the edges of the field, as crosses are relatively easy to defend against, and Klopp’s strategy of defending deep in the opponent’s half, a tactic that has become popular due to its effectiveness, known as gegenpressing. Another strategy is to focus on set pieces like corners and throw-ins, which are often considered secondary to regular play but can potentially result in a significant number of goals.
As data science has advanced, many metrics like expected goals have been realized to be too simplistic, eg: expected goals only consider the position of the shot and not the direction, the defense, or other factors. This realization has led to the development of more complex metrics that take into account the direction of the shot, the position of the players during a pass, and not just the number of passes, as well as the position of the defense during a shot. One such advanced metric is called packing, which measures the number of times a defender is bypassed and is strongly correlated with winning. However, it requires a lot of labor to annotate video data. Mathematical methods like the use of Voronoi diagrams to assess space control and other sophisticated data science techniques are constantly being developed and presented at conferences.
Data based methods are also used to scout for new players. The difficulty lies in assessing how good a player is when they are at the top of a lower league – whether they display top talen, and when a player’s talent is not evident due to being on a bad team. This is important to get right because bad deals cost a lot of money for teams when they acquire a mediocre player for a large sum, overestimating them because of a lucky streak. Data science can prevent this by identifying weaknesses in these players prior to acquisition The goal impact metric tries to quantify a player’s impact on the game, even if they don’t make obvious moves like shooting on goals or blocking passes.
The last section of the book is about data-based methods to develop special apps to train players’ decision-making capabilities on the field, and also to create personality profiles so coaches can understand how they are motivated differently. Nowadays, analytics-driven football has effectively become mainstream, and metrics like expected goals are often taken into consideration for evaluating players’ and coaches’ performances rather than just the actual number of goals or games won. There are many startups in this space trying to improve analytics methods, and some teams, like Barcelona, strive to be on the cutting edge and submit their work to deep learning conferences.